Understanding the Importance of Labeling Training Data
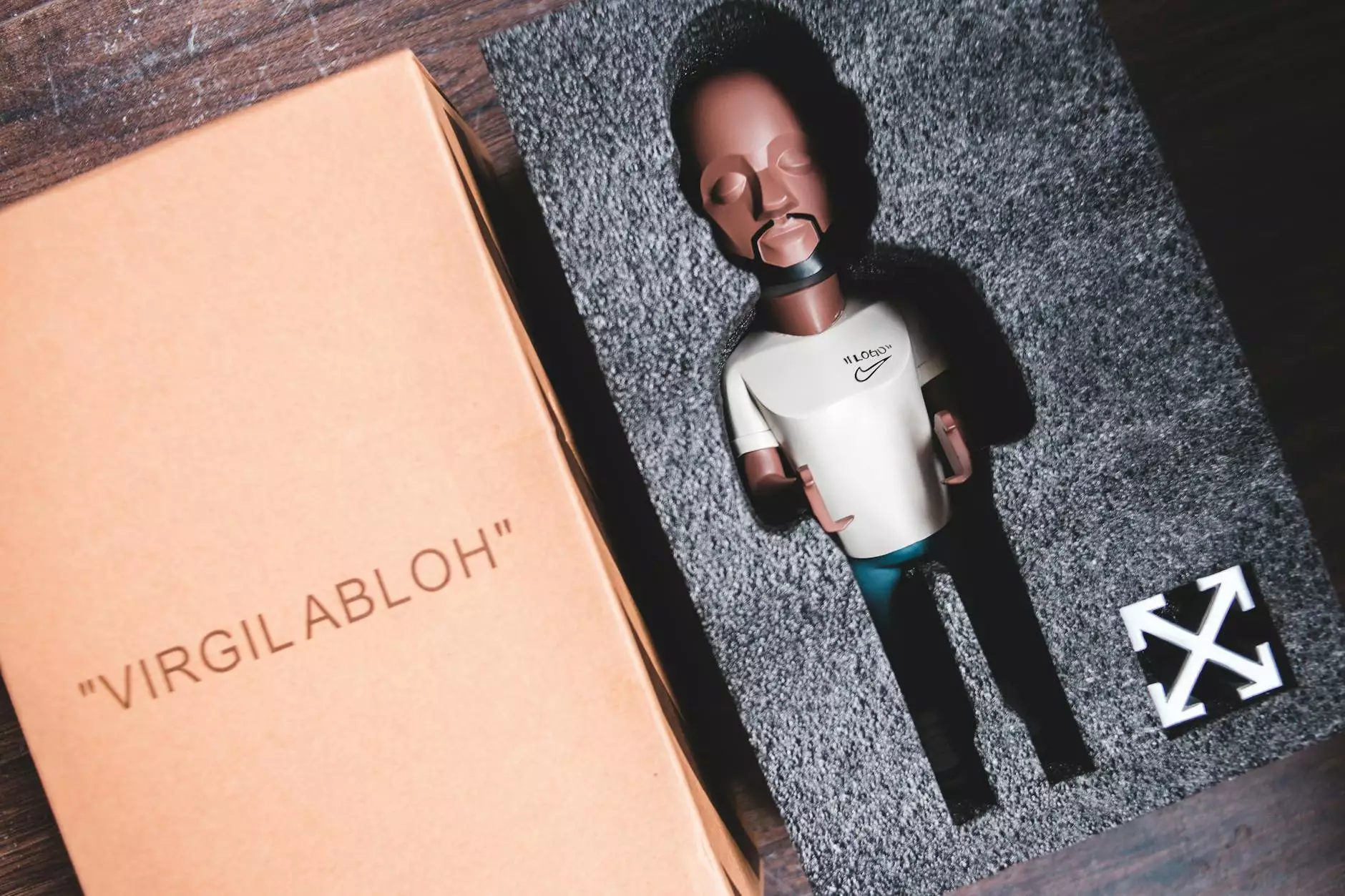
In today's digital age, where data reigns supreme, labeling training data plays a crucial role in the development of effective machine learning models. The effectiveness and accuracy of AI models hinge on the quality of the labeled data available for training. This article delves deep into the significance of data annotation, the various types of data annotation tools available, and how platforms like KeyLabs.ai are revolutionizing the data annotation landscape.
The Foundation of Machine Learning: What is Labeling Training Data?
Labeling training data involves the process of identifying and categorizing data to train machine learning algorithms. The process entails:
- Data Collection: Gathering relevant data from various sources.
- Annotation: Applying labels to the collected data to define its features.
- Training: Feeding the labeled data into machine learning models to help them learn and make predictions.
Without the precise labeling of data, machine learning models cannot learn effectively, leading to subpar performance in real-world applications.
Why Labeling Training Data Matters
The significance of labeling training data cannot be overstated for several reasons:
1. Enhances Model Performance
Models trained on well-labeled datasets exhibit higher accuracy, reliability, and robustness. The quality of the labels directly impacts the model's ability to recognize patterns and make informed decisions.
2. Reduces Bias
Labeling training data accurately helps in minimizing the risk of bias in AI models. When data is annotated thoughtfully, incorporating diverse perspectives, the models are more likely to perform fairly across different demographics and scenarios.
3. Facilitates Clear Communication
Incorporating precise labels makes it easier for teams to understand the data context and model functionalities. This clear communication is vital for collaboration among project stakeholders.
4. Supports Compliance and Ethical Standards
With increasing regulations around AI and data usage, having adequately labeled data ensures compliance with standards such as GDPR. Proper labeling practices support ethical AI development.
Different Types of Data Annotation Tools
Choosing the right data annotation tool is critical for efficient labeling of training data. Here are the primary tools used in the industry:
1. Image Annotation Tools
These tools are designed to label visual data, which is essential for computer vision applications. Techniques include:
- Bounding Boxes: Drawing rectangles around objects in images.
- Segmentation: Dividing images into segments to identify regions of interest.
- Keypoint Annotation: Marking specific points on an image that represent crucial features.
2. Text Annotation Tools
Text annotation focuses on labeling textual data, which is vital for Natural Language Processing (NLP) tasks. Annotation types include:
- Named Entity Recognition: Identifying and classifying entities in text.
- Sentiment Analysis: Marking text as positive, negative, or neutral.
- Text Classification: Assigning predefined categories to text segments.
3. Audio and Video Annotation Tools
These tools label audio and video data, crucial for applications such as voice recognition and video analysis. Key techniques include:
- Speech Recognition: Transcribing spoken words into text.
- Activity Recognition: Identifying and labeling actions in videos.
KeyLabs.ai: Your Go-To Data Annotation Platform
KeyLabs.ai is at the forefront of offering a comprehensive suite of data annotation tools, facilitating the efficient labeling of training data across various domains. Here’s why KeyLabs.ai stands out:
1. User-Friendly Interface
The intuitive interface of the KeyLabs.ai platform allows users to annotate data effortlessly, reducing the learning curve for new users and increasing productivity.
2. Scalability
As your data needs grow, so does KeyLabs.ai. The platform can easily handle large volumes of data, ensuring you can keep up with the demands of your AI projects.
3. Quality Assurance
Maintaining high-quality data is essential. KeyLabs.ai implements rigorous quality control measures to ensure that all labeled data meets the highest industry standards.
4. Customizable Solutions
Understanding that each business is unique, KeyLabs.ai offers customizable annotation solutions tailored to specific project needs, enabling more relevant and precise labeling.
Best Practices for Labeling Training Data
To maximize the effectiveness of data annotation efforts, consider the following best practices:
1. Define Clear Guidelines
Establish clear and comprehensive guidelines for annotators to follow. This reduces variability in annotations and enhances the consistency of the labeled data.
2. Invest in Training
Providing training for annotators ensures they understand the importance of precision in labeling. Continuous feedback can help improve their skills over time.
3. Use Quality Control Mechanisms
Implement regular audits and quality checks to ensure that the labeled data remains accurate and reliable. Feedback loops are essential for ongoing improvement.
4. Foster Collaboration
Encouraging collaboration among team members enhances knowledge sharing and can lead to better-crafted labels through diverse expertise.
The Future of Labeling Training Data
The landscape of data annotation is evolving. As machine learning and AI continue to grow, the demand for high-quality labeled data will only increase. Innovations such as:
- Automated Annotation Tools: These use AI to accelerate the labeling process, while still allowing for human oversight.
- Batch Processing: Tools that allow for batch processing of large datasets significantly reduce the time required for labeling.
- Active Learning: This approach involves the model requesting labels for uncertain predictions, thereby improving efficiency.
Conclusion: Unlock the Power of Your Data with KeyLabs.ai
The journey to successful AI implementation starts with effective labeling training data. By leveraging platforms like KeyLabs.ai, businesses can enhance their AI projects, ensuring that their models are robust, accurate, and effective in real-world applications. Investing in quality data labeling is an investment in the future of your business.
Whether you are initiating a new project or looking to improve an existing model, remember that the quality of your training data is paramount – and with the right tools and practices, you can achieve excellence in your AI endeavors.